Nightshade Data Poisoning Tool Could Help Artists Fight AI
The potent Nightshade targets text-to-image generators.
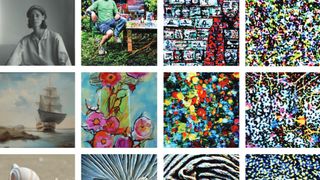
A new image data tool dubbed Nightshade has been created by computer science researchers to "poison" data meant for text-to-image models. Nightshade adds imperceptible changes to creators’ images to uploaded online. If a data scraper subsequently adds one of these altered images to its data set, it will “introduce unexpected behaviors,” into the model, poisoning it.
A preview of a paper called Prompt-Specific Poisoning Attacks on Text-to-Image Generative Models was recently published on arXiv, outlining the scope and functionality of Nightshade. MIT Technology Review has gained some further insight from talks with the team behind the project.
The issue of AI models being trained on data without explicit creator permission, and then ‘generating new data’ based on what it has learned, has been well covered in news in recent months. What appears to be a data scraping free-for-all followed by machine regurgitation, with little or no attribution, has caused creators from many disciplines to frown upon current generative AI projects.
Nightshade is intended to help visual artists, or at least make AI data scrapers more wary about grabbing data willy-nilly. The tool is capable of poisoning image data with no detriment to human viewers, but will disrupt AI models used by generative tools like DALL-E, Midjourney, and Stable Diffusion.
Some example data poisoning effects of Nightshade could include: dogs becoming cats, cars becoming cows, cubism becoming anime, hats becoming toasters, and other chaos. See below for examples of the corrosive effects of Nightshade in action.
Previously it was thought that poisoning a generative AI might need millions of poison samples pushed towards the training pipeline. However, Nightshade is claimed to be a “prompt-specific poisoning attack... optimized for potency.” Quite startlingly, Nightshade can do its disruptive business in under 100 poison samples. Moreover, the researchers say that Nightshade poison effects "bleed through" to related concepts, and multiple attacks can composed together in a single prompt. The end result is to disable the generation of meaningful images based on user text prompts.
The researchers don’t intend tools like Nightshade to be used without due care. Rather it is intended “as a last defense for content creators against web scrapers that ignore opt-out/do-not-crawl directives.” Tools like this could become a deterrent against the Wild West-era AI data scrapers. Even now, flagship AI companies like Stability AI and OpenAI are only omitting artworks when users jump through hoops to opt-out, according to MIT Technology Review's report. Some think it should instead be the AI companies that are required to chase users to opt-in.
Stay On the Cutting Edge: Get the Tom's Hardware Newsletter
Get Tom's Hardware's best news and in-depth reviews, straight to your inbox.
Ben Zhao, a professor at the University of Chicago who led the Nightshade research team, is also behind Glaze, a tool that allows creators to mask their personal art style to prevent it being scraped and mimicked by AI companies. Nightshade, which is being prepared as an open-source project, could be integrated into Glaze at a later date to make AI data poisoning an option.
Mark Tyson is a news editor at Tom's Hardware. He enjoys covering the full breadth of PC tech; from business and semiconductor design to products approaching the edge of reason.
-
setx Again this nonsense of "imperceptible changes to creators’ images".Reply
I'd bet it either makes images look significantly synthetic or the effect can be completely removed by simple gaussian/median filter (that is transparent for humans/learning given sufficient input resolution). -
Co BIY The big aggregators (and state actors) already took all the data and have a safe copy stored for later use.Reply
Steal now, decrypt later. Bet they did the same with alla that.
Archived images and text may be crucial for future generative AI to avoid feeding the model not-only poisoned data but AI generated data (AI poisoned data?) which may magnify or concentrate bad effects like in-breeding or biomagnification in a food chain. -
bit_user
You really ought to read the paper. As usual, the article has a link (which goes to the arxiv.org page and that has a PDF link). I'll just say I was very impressed by not only how thorough the authors were in anticipating all of the applications and possible countermeasures against the technique, but also various scenarios and their consequences.setx said:Again this nonsense of "imperceptible changes to creators’ images".
I'd bet it either makes images look significantly synthetic or the effect can be completely removed by simple gaussian/median filter (that is transparent for humans/learning given sufficient input resolution).
It's one of those papers you need to spend a bit of time with. They don't give away the goods on the technique until about section 5, so don't think you can just read the abstract, conclusion, and glance at some results.
As impressed as I was with the research, I was even more disturbed by their findings, because it indeed seems truly imperceptible and very destructive to models - especially when multiple such attacks are independently mounted. Not only that, but it seems exceptionally difficult to defend against. Perhaps someone will find an effective countermeasure, but I came away with the impression that an analogous attack against spy satellites would be to fill low-earth orbit with a massive amount of debris. That's because it's both indiscriminate and, like a good poison, you don't need very much of it.
What it's not is a protection mechanism like Glaze:
https://www.tomshardware.com/news/glaze-1-modifies-images-to-protect-art
They do outline a scenario in which a company might use it to protect their copyright characters, but you couldn't use it to protect individual artworks. -
bit_user
Eh, yes and no. They reference one opensource data set, called LAION-Aesthetic, which:Co BIY said:The big aggregators (and state actors) already took all the data and have a safe copy stored for later use.
Steal now, decrypt later. Bet they did the same with alla that.
Archived images and text may be crucial for future generative AI to avoid feeding the model not-only poisoned data but AI generated data (AI poisoned data?) which may magnify or concentrate bad effects like in-breeding or biomagnification in a food chain.
"is a subset of LAION-5B, and contains 600 million text/image pairs and 22833 unique, valid English words across all text prompts"
So, you can even access such datasets if you're not one of the big guys. They also reference other datasets.
The problem with not taking in any new data is that your models never advance beyond 2022, or whenever generative imagery started being published en masse. Your models would never learn how to generate images of people, places, or things newer than that. Still, I'm with you on using archived and sequestered data for older imagery, so as to minimize exposure to such exploits. For everything else, establishing chain-of-custody will be key.