Nvidia shows off Rubin Ultra with 600,000-Watt Kyber racks and infrastructure, coming in 2027
Planning for the future with up to 600kW per rack.
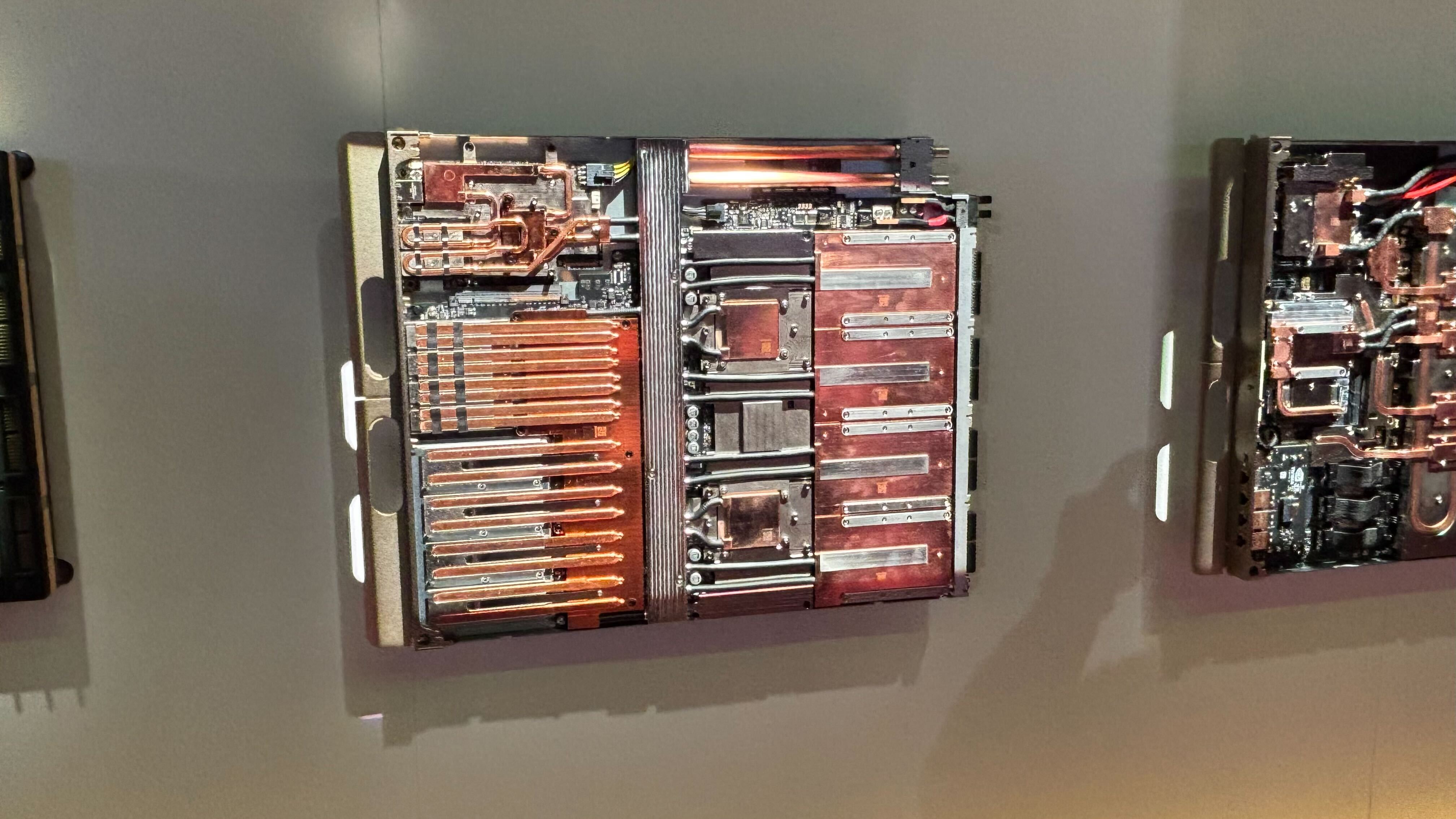
Nvidia showed off a mockup of its future Rubin Ultra GPUs with the NVL576 Kyber racks and infrastructure at GTC 2025. These are intended to ship in the second half of 2027, more than two years away, and yet, as an AI infrastructure company, Nvidia is already well on its way to planning how we get from where we are today to where it wants us to be in a few years. That future includes GPU servers that are so powerful that they consume up to 600kW per rack.
The current Blackwell B200 server racks already use copious amounts of power, up to 120kW per rack (give or take). The first Vera Rubin solutions, slated for the second half of 2026, will use the same infrastructure as Grace Blackwell, but the next Rubin Ultra solutions intend to quadruple the number of GPUs per rack. Along with that, we could be looking at single rack solutions that consume up to 600kW, as Jensen Huang verified during a question-and-answer session, with full SuperPODS requiring multi-megawatts of power.
Kyber is the name of the rack infrastructure that will be used for these platforms.
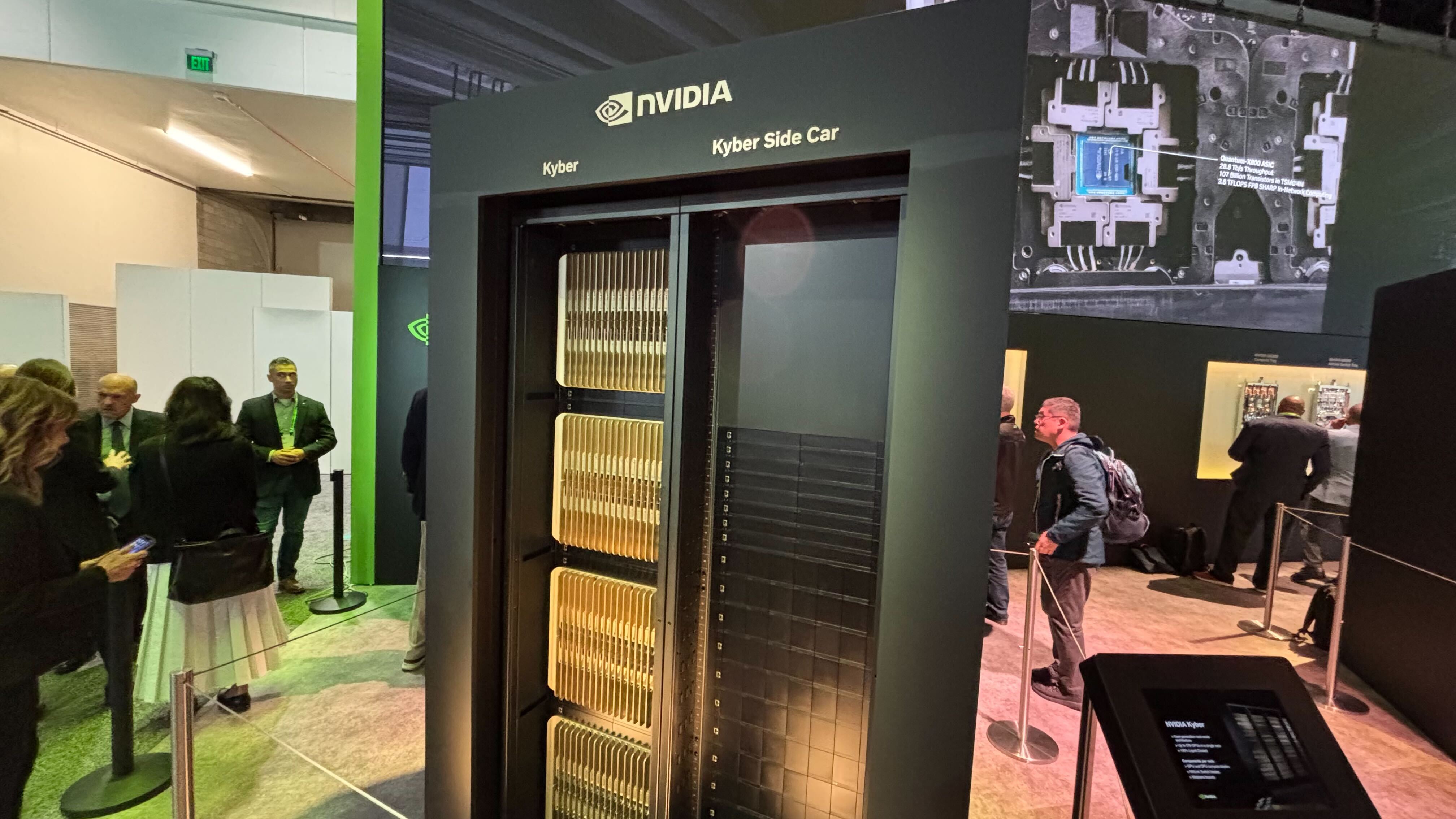
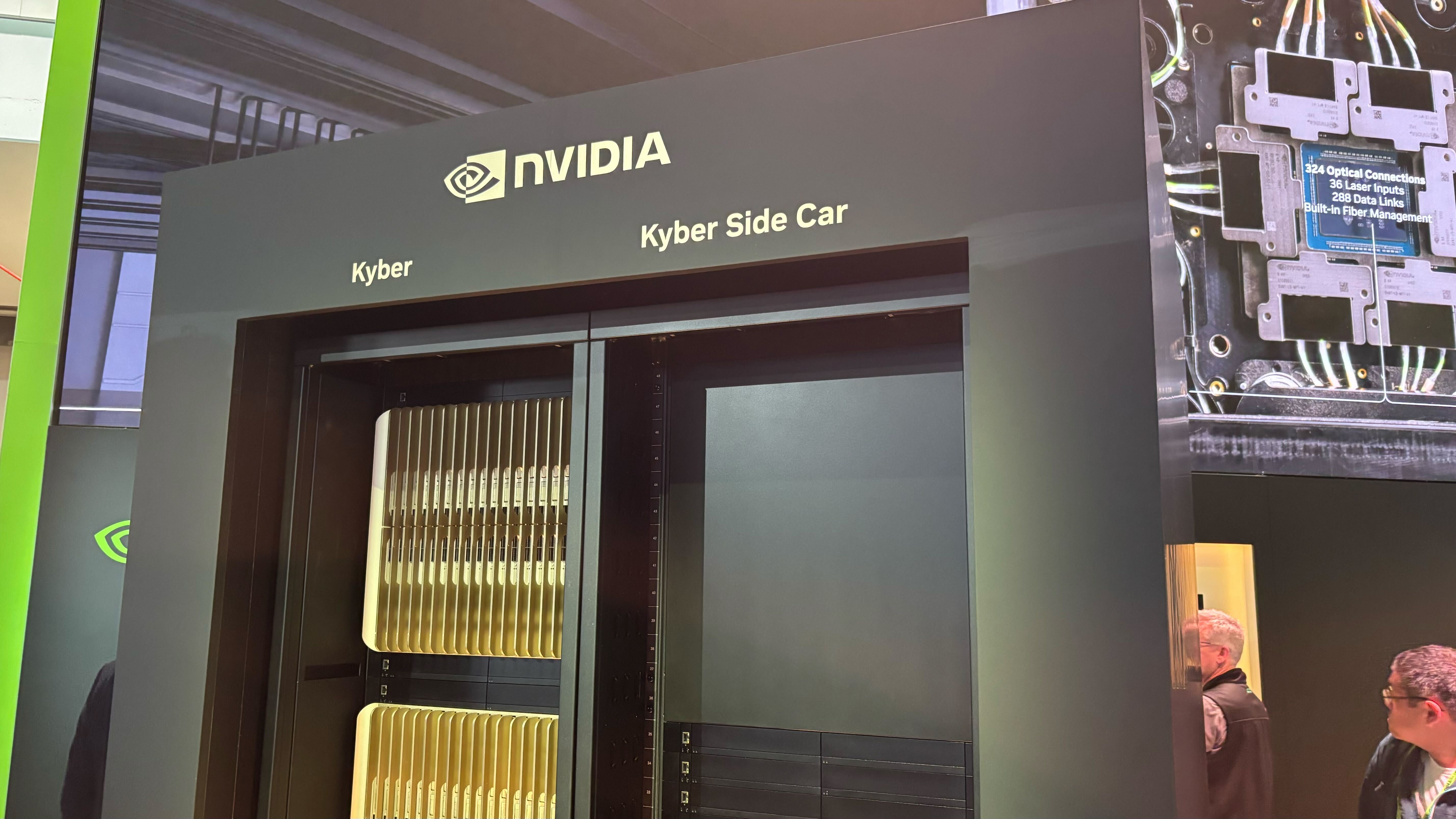
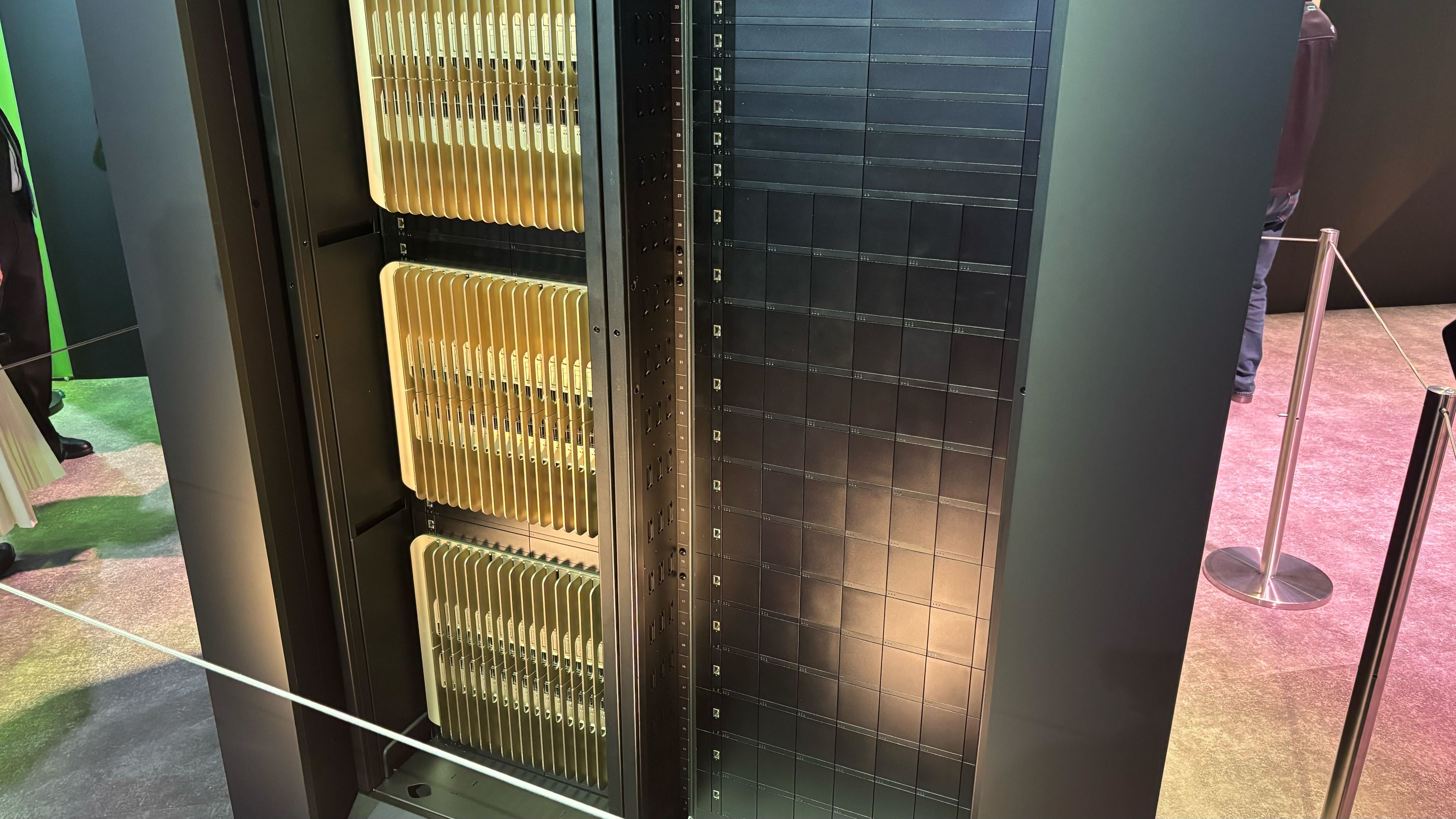
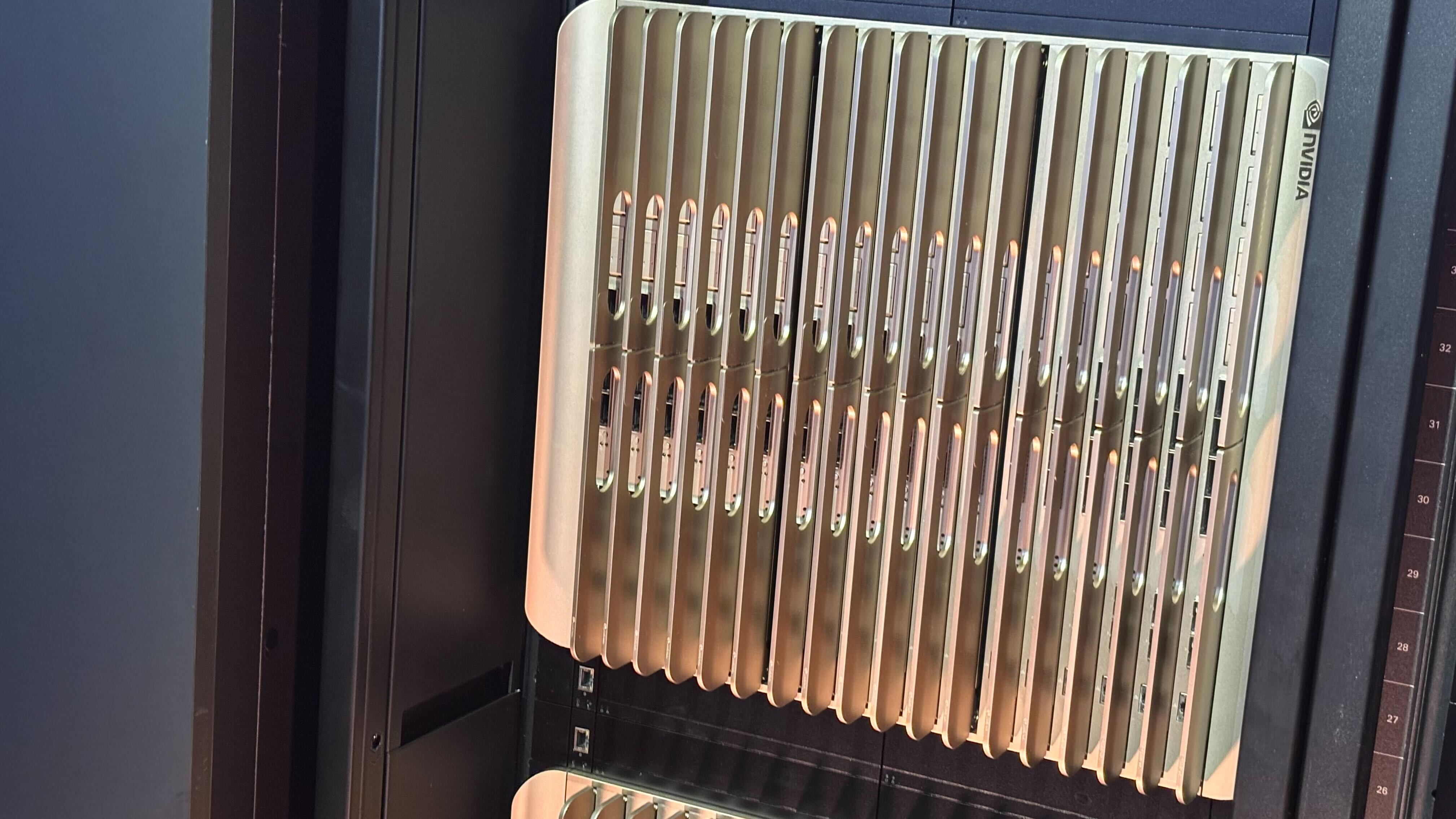
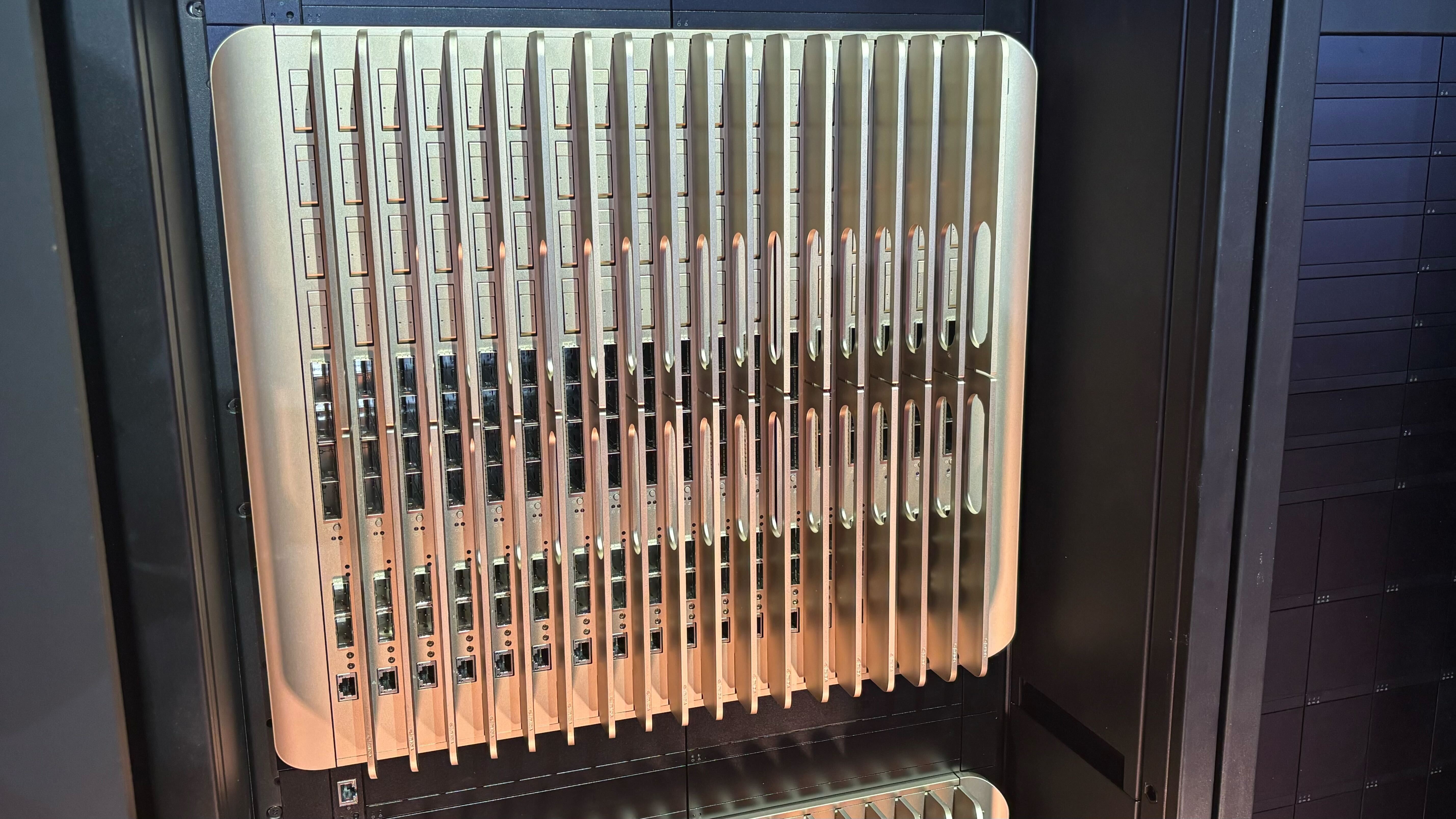
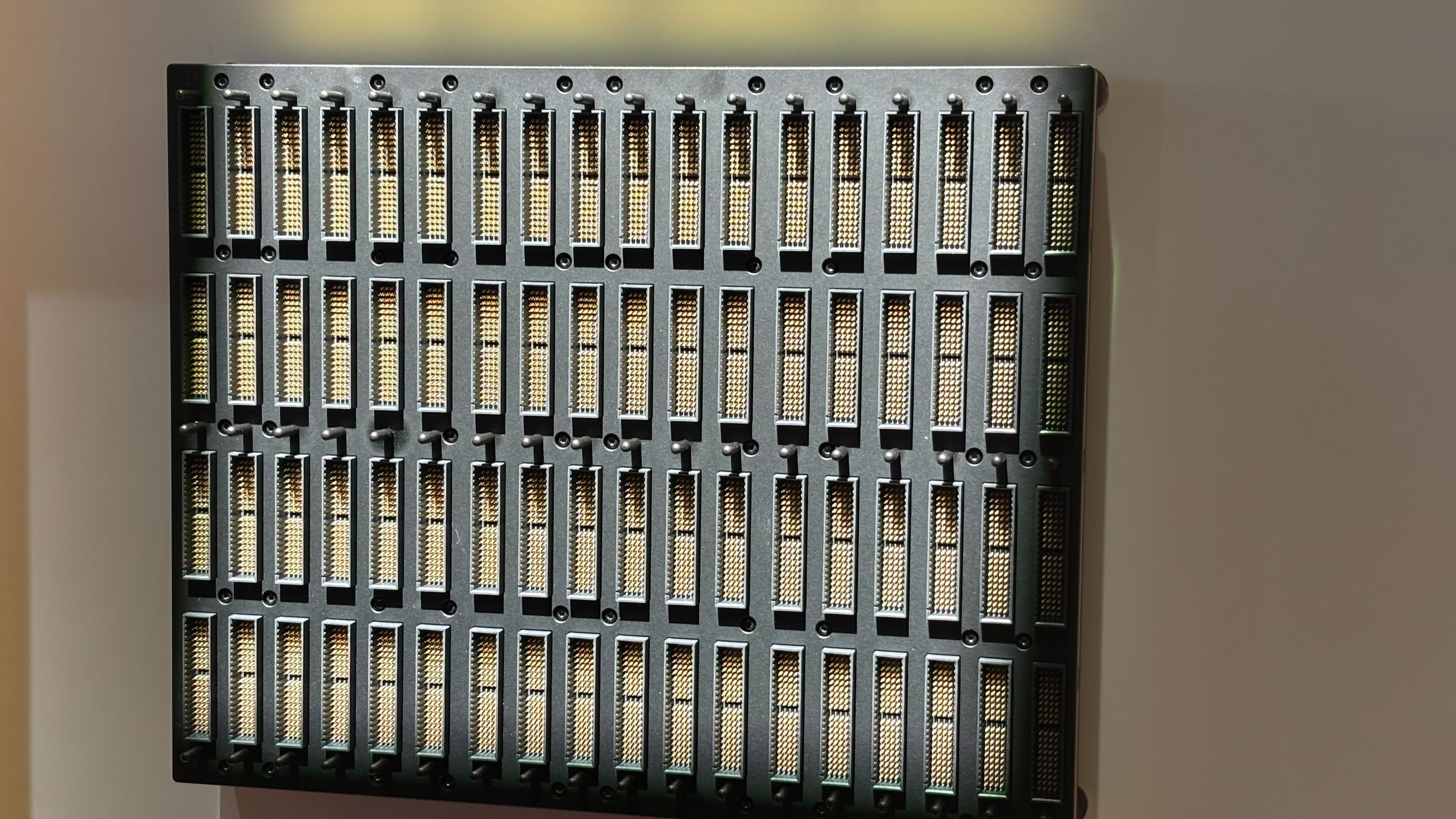
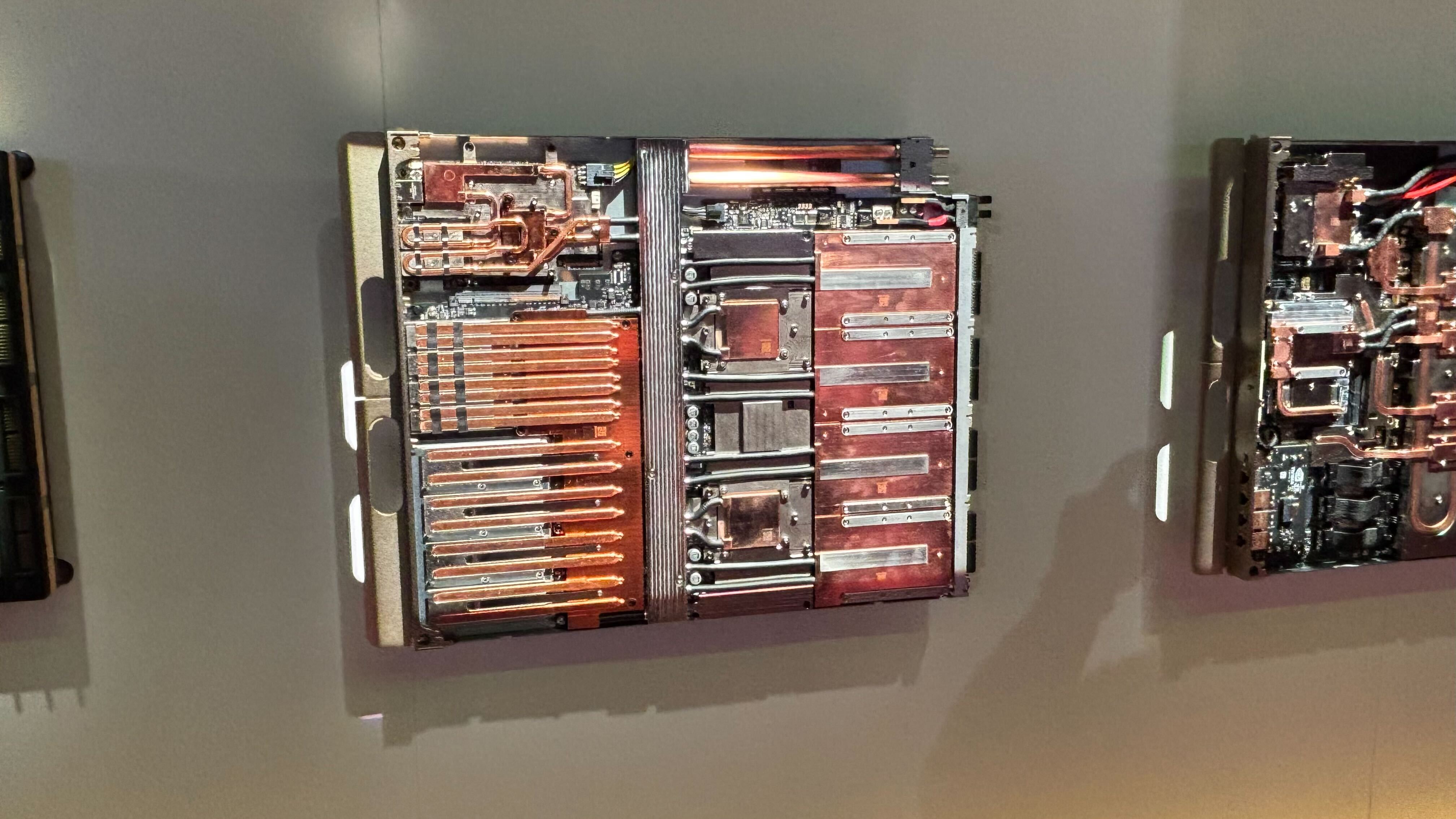
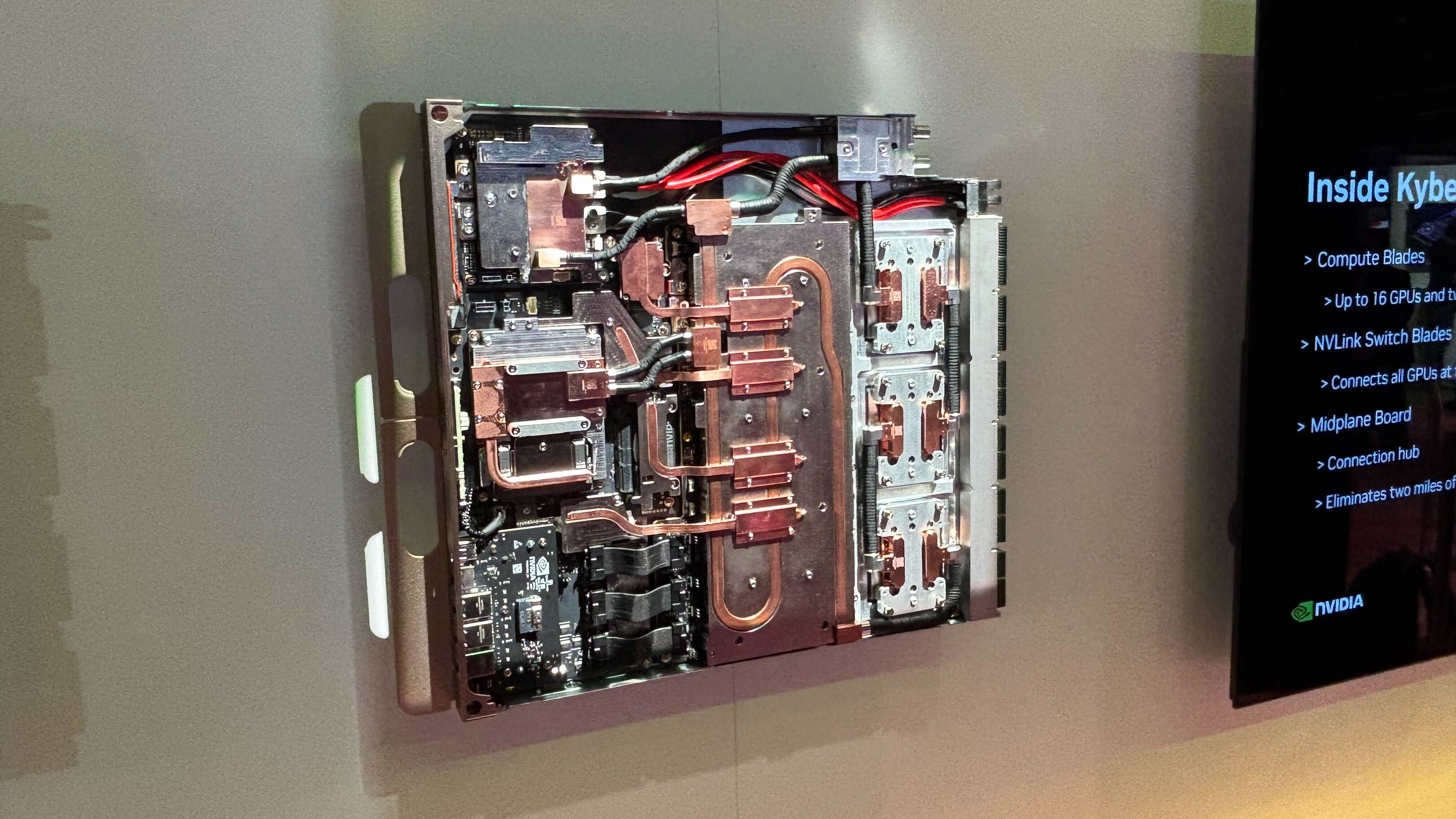
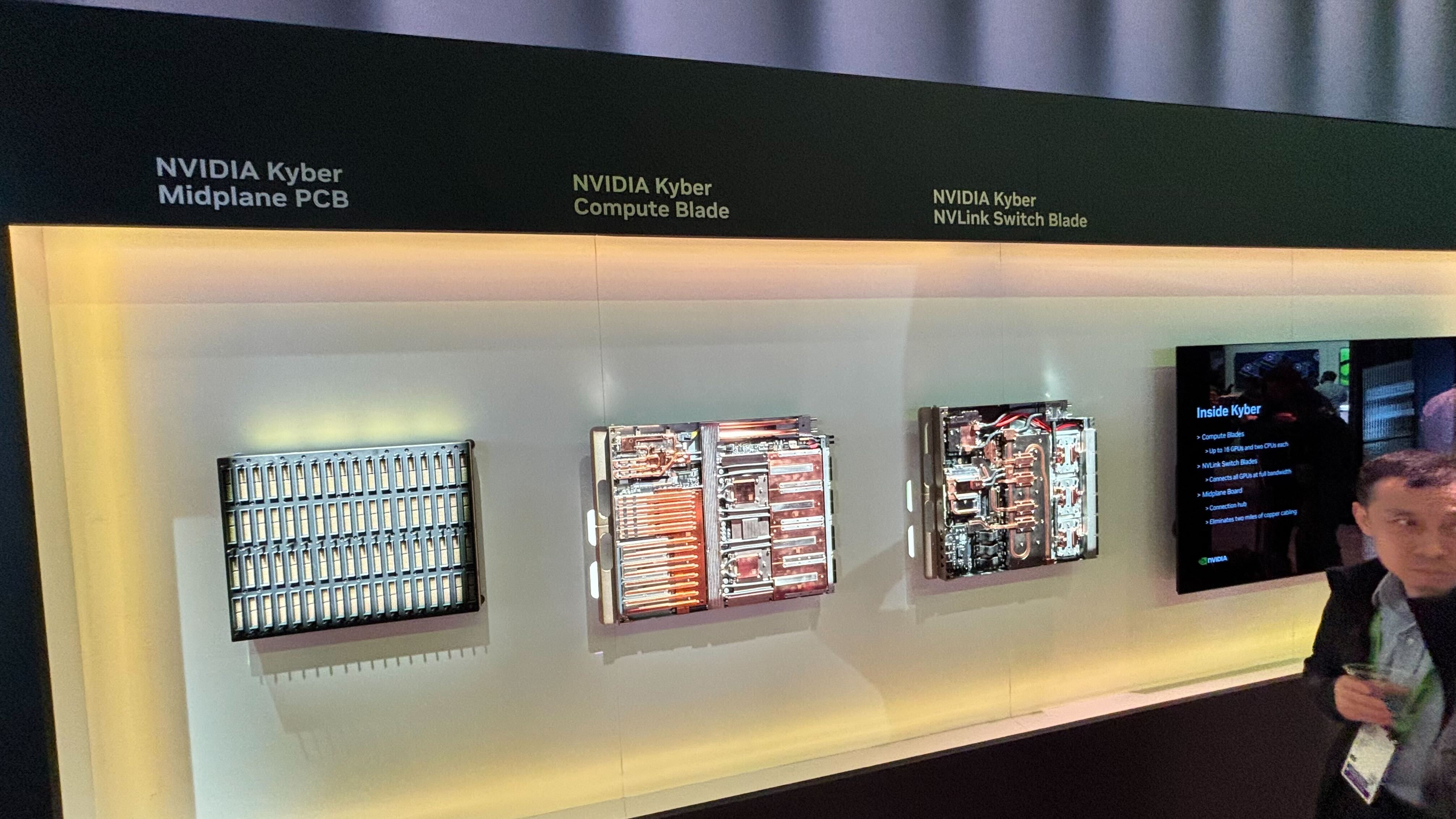
There are no hard specifications yet for Rubin Ultra, but there are performance targets. As discussed during the keynote and in regards to Nvidia's data center GPU roadmap going beyond Blackwell Ultra B300, Rubin NVL144 racks will offer up to 3.6 EFLOPS of FP4 inference in the second half of next year, with Rubin Ultra NVL576 racks in 2027 delivering up to 15 EFLOPS of FP4. It's a huge jump in compute density, along with power density.
Each Rubin Ultra rack will consist of four 'pods,' each of which will deliver more computational power than an entire Rubin NVL144 rack. Each pod will house 18 blades, and each blade will support up to eight Rubin Ultra GPUs — along with two Vera CPUs, presumably, though that wasn't explicitly stated. That's 176 GPUs per pod, and 576 per rack.
The NVLink units are getting upgrades as well and will each have three next-generation NVLink connections, whereas the current NVLink 1U rack-mount units only have two NVLink connections. Either prototypes or mockups of both the NVLink and Rubin Ultra blades were on display with the Kyber rack.
No one has provided clear power numbers, but Jensen talked about data centers in the coming years potentially needing megawatts of power per server rack. That's not Kyber, but whatever comes after could very well push beyond 1MW per rack, with Kyber targeting around 600kW if it keeps with the current 1000~1400 watts per GPU of the Blackwell series.
Stay On the Cutting Edge: Get the Tom's Hardware Newsletter
Get Tom's Hardware's best news and in-depth reviews, straight to your inbox.
Jarred Walton is a senior editor at Tom's Hardware focusing on everything GPU. He has been working as a tech journalist since 2004, writing for AnandTech, Maximum PC, and PC Gamer. From the first S3 Virge '3D decelerators' to today's GPUs, Jarred keeps up with all the latest graphics trends and is the one to ask about game performance.
-
bit_user
So, what's the energy-density of say... an aluminum smelter?The article said:whatever comes after could very well push beyond 1MW per rack
: D
P.S. I'd love to see the home gaming PCs of whoever designed these water-cooling setups:
So, how does it work, when you want to pull one of those blades out of a rack? Is there like a valve you hit that first blows pressurized air into the tubing, so that water doesn't go everywhere when you yank it out? -
Flayed Maybe we will be able to buy graphics cards once they run out of power generation capacity :PReply -
edzieba
QD-fittings, same as we've been using since watercooling was fully custom with DIY blocks and car radiators (that had to sit outside the chassis).bit_user said:So, how does it work, when you want to pull one of those blades out of a rack? Is there like a valve you hit that first blows pressurized air into the tubing, so that water doesn't go everywhere when you yank it out? -
JarredWaltonGPU
The word on the street is that companies are getting paid $1 per million tokens generated. I don't know if that's accurate, but a traditional LLM might generate 500~1000 tokens for a response. A reasoning model (DeepSeek R1-671B is given as the example) can require 15~20 times more tokens per response. So, not only does it cost more to run, but it needs more hardware to run faster, and presumably people (companies, mostly) would pay more to access its features.Alex/AT said:Lots of power for no viable return.
How many tokens per day can an NVL72 rack generate? Seems like Nvidia is saying 5.8 million TPS per MW. With a single NVL72 using about 125kW, that would mean 0.725 TPS roughly. Or 62,640 million tokens per day. Which at $1 per million would be nearly $63K. Minus power costs and other stuff, let's just call it $60K per day potential income?
That would be a potential ROI of maybe 75 days, give or take, if you're able to run that flat out 24/7. It's unlikely to be sustained, but I do think there's a lot more investment here than any of the gamers think. Hundreds of billions are going into AI per year, and that could scale to trillions per year.
And tokens aren't just about ChatGPT. Robotics and image generation and video generation and more can all distill down to tokens per second. Financial analysts will use AI to help model things and decide when to buy/sell. Doctors and the medical field will use AI. Cars will use AI. Our phones already use AI. The list of where AI is being used is pretty much endless.
Jensen has said, repeatedly, that data centers are becoming power constrained. So now it's really a question of tokens per watt, or tokens per MW. And most process node improvements are only giving maybe 20% more efficiency by that metric, while software and architectural changes can provide much greater jumps.
As with so many things, we'll need to wait and see. Does AI feel like a bubble? Sure. But how far will that bubble go? And will it every fully pop? Bitcoin has felt like far more of a bubble for 15 years now, but it keeps going. I would not bet against Nvidia and Jensen, personally. Gelsinger saying Nvidia got "lucky" is such BS, frankly... sour grapes and lack of an ability to push through important projects. Larrabee could have gone somewhere, if Gelsinger and Intel had been willing to take the risk. But it went against the CPU-first ethos and so it didn't happen. -
bit_user
Yeah, the only minor quibble I have is that Gelsinger needed to do more than just "push through". I mean, they did actually just forge ahead with Xeon Phi using x86 cores. What they needed to do was design the best architecture for the task, not slap together a Frankensteinian card with spare parts from the bin (which they literally did, to some extent - first using Pentium P54C cores, and then using Silvermont Atom cores).JarredWaltonGPU said:I would not bet against Nvidia and Jensen, personally. Gelsinger saying Nvidia got "lucky" is such BS, frankly... sour grapes and lack of an ability to push through important projects. Larrabee could have gone somewhere, if Gelsinger and Intel had been willing to take the risk. But it went against the CPU-first ethos and so it didn't happen.
Instead, they assumed the HPC market would prioritize ease of programmability & legacy compatibility above all else, in spite of many decades of history, where the HPC market embraced some fairly odd and esoteric computing architectures. The big difference between the HPC market vs. traditional computers is that the upside of deviating from a mainstream CPU was much bigger than for something like your desktop PC. Enough to justify porting or rewriting some of the software. Again, there's a history of that, so it's not like they couldn't have predicted such an outcome.
At the time, I was following Xeon Phi and about the closest it ever came to Nvidia, on paper, was about a factor of 2 (i.e. half the performance) of Nvidia's datacenter GPUs' fp64 TFLOPS. However, what I've read from people who actually tried to program Xeon Phi is that you couldn't get anywhere near its theoretical performance. So, the reality might've been that they were closer to being behind by like a factor of 5 or 10. And that was with a bit of effort.
I'll bet Intel thought at the time that, "if you build it, they will come" has an extremely poor track record, in computing. Thus, simply making a better architecture is no guarantee of its success. However, Nvidia didn't simply build GPUs for HPC - they aggressively pushed CUDA and shoved free & discounted hardware on lots of universities and grad students. It's no accident that AI researchers embraced CUDA. Nvidia didn't wait for them to come knocking, Jensen went out and found them. -
JarredWaltonGPU
Yeah, absolutely. Every time Gelsinger trots out Larrabee, I tend to think, "Yeah, but..." and all of the stuff you've listed above. Larrabee was a pet project that in truth wasn't designed well. It was a proof of concept that just didn't interest the Intel executives and board at the time. Which is why I say the "luck" stuff is a bunch of BS.bit_user said:Yeah, the only minor quibble I have is that Gelsinger needed to do more than just "push through". I mean, they did actually just forge ahead with Xeon Phi using x86 cores. What they needed to do was design the best architecture for the task, not slap together a Frankensteinian card with spare parts from the bin (which they literally did, to some extent - first using Pentium P54C cores, and then using Silvermont Atom cores).
Instead, they assumed the HPC market would prioritize ease of programmability & legacy compatibility above all else, in spite of many decades of history, where the HPC market embraced some fairly odd and esoteric computing architectures. The big difference between the HPC market vs. traditional computers is that the upside of deviating from a mainstream CPU was much bigger than for something like your desktop PC. Enough to justify porting or rewriting some of the software. Again, there's a history of that, so it's not like they couldn't have predicted such an outcome.
At the time, I was following Xeon Phi and about the closest it ever came to Nvidia, on paper, was about a factor of 2 (i.e. half the performance) of Nvidia's datacenter GPUs' fp64 TFLOPS. However, what I've read from people who actually tried to program Xeon Phi is that you couldn't get anywhere near its theoretical performance. So, the reality might've been that they were closer to being behind by like a factor of 5 or 10. And that was with a bit of effort.
I'll bet Intel thought at the time that, "if you build it, they will come" has an extremely poor track record, in computing. Thus, simply making a better architecture is no guarantee of its success. However, Nvidia didn't simply build GPUs for HPC - they aggressively pushed CUDA and shoved free & discounted hardware on lots of universities and grad students. It's no accident that AI researchers embraced CUDA. Nvidia didn't wait for them to come knocking, Jensen went out and found them.
Nvidia has worked very hard to get where it is. AI didn't just fall into Nvidia's lap haphazardly. As you say, Nvidia went out and found customers. More than that, it saw the potential for AI right when AlexNet first came out, and it hired all those researchers and more to get ahead of the game. If AlexNet could leapfrog the competition for image classification back then, where might a similar approach gain traction? That was the key question and one Nvidia invested heavily into answering, with both hardware and software solutions.
On a related note: Itanium. That was in theory supposed to be a "from the ground up" design for 64-bit computing. The problem was that Intel again didn't focus on creating the best architecture and design. Or maybe it did try to do that and failed? But too often Intel has gotten caught with its feet in two contradictory worlds. You can't do x86 on one hand and a complete new "ideal" architecture on the other hand and have them work together perfectly. Larrabee was the same story for GPUs: x86 on the one hand due to "familiarity" with GPU-like aspects on the other hand for compute.
What's interesting is to hear about where CUDA started. I think Ian Buck was the main person, and he had a team of like four people maybe. He said something about that in a GTC session yesterday. It started so small, but it was done effectively and ended up becoming the dominant force it is today. -
Jame5 That future includes GPU servers that are so powerful that they consume up to 600kW per rack.
That future includes GPU servers that are so power hungry that they consume up to 600kW per rack.
Small typo in that sentence.
We are seriously in the 1950's automotive era of performance in computing right now. Bigger and more power hungry by any means necessary, efficiency be damned. Throwing more power at something is not the same as making it better. It goes 1mph faster than last year's model, what does it matter if it gets half the fuel efficiency? -
JarredWaltonGPU
No, we're really not. We've hit the end of Dennard scaling and easy lithography upgrades. A 20% improvement in performance per watt is all we can expect from the hardware, on its own, so now we need better architectures and software algorithms.Jame5 said:That future includes GPU servers that are so power hungry that they consume up to 600kW per rack.
Small typo in that sentence.
We are seriously in the 1950's automotive era of performance in computing right now. Bigger and more power hungry by any means necessary, efficiency be damned. Throwing more power at something is not the same as making it better. It goes 1mph faster than last year's model, what does it matter if it gets half the fuel efficiency?
The reason anyone is even willing to consider 600kW racks is that companies need the compute. We have entered a new era of needing way more compute than we currently have available. And if you look at performance per watt, all the new Blackwell Ultra B300, Rubin, and Rubin Ultra aren't just adding more compute and scaling to higher power; they're more efficient.
Rubin Ultra is basically 4X the number of GPUs per rack. So yes, that's 4X the power (and then some), but 6X the performance. If you increase power by 20% and increase performance by 50%, that's better efficiency, which is not the same as being power hungry.
Could you cut power use? Sure. Maybe with lower clocks and voltages you cut performance 30% and reduce power 40% or something. But a ton of the power is going to memory and interchip communications, not just to the GPU cores. So I'm not sure you really can get the same efficiency gains by running these data center chips at lower clocks and voltages as you'd get if we were talking about single GPUs.