Jensen says solving AI hallucination problems is 'several years away,' requires increasing computation
The CEO also talked about how much AI computing power increased in the past 10 years and Nvidia’s single greatest contribution to AI.
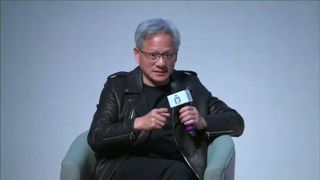
Nvidia CEO Jensen Huang recently said he doesn't think the industry can solve the AI hallucination issue, wherein an AI makes up information to fill in its knowledge gaps, for several more years. Huang also outlined how he had wooed his wife by promising he would be a CEO by the age of 30. Huang discussed these and other topics, like how much computing needs increased in the past 10 years, during an interview at the Hong Kong University of Science and Technology.
The host said the demand for AI computing power jumps four times annually. “In 10 years, it’s [an] enormous number, actually a million.” He also added that’s why Nvidia’s stock went up 300x. “The computing needs are a million times more, so then it explains the stock probably is not that expensive.” He then asked Huang, “As you look into the future with your crystal ball, are we going to see that a million times more needs increase for the next 10 years?”
Huang did not give a direct answer, but he first explained how artificial intelligence is being developed today. The first area is pre-training, which the Nvidia CEO compared to going to college. Here, the AI takes in all the data in the world and discovers knowledge through it. Huang says this is a “very important milestone, but it’s not enough.” The second area in the AI development process is post-training, where it dives deep into a particular skill via “reinforcement learning [through] human feedback, reinforcement learning [through] AI feedback, synthetic data generation, multipath learning, reinforcement learning [sic]—there’s a whole bunch of techniques.”
Finally, the third area of AI development is Test Time Scaling, which he also referred to as “thinking.” This is much more complicated, as the AI now breaks the problem down step by step to find a solution. It might have to iterate and sometimes simulate various outcomes, especially if the answer is not predictive. He says, “We call that thinking, and the longer you think, maybe the higher quality the answer would become.”
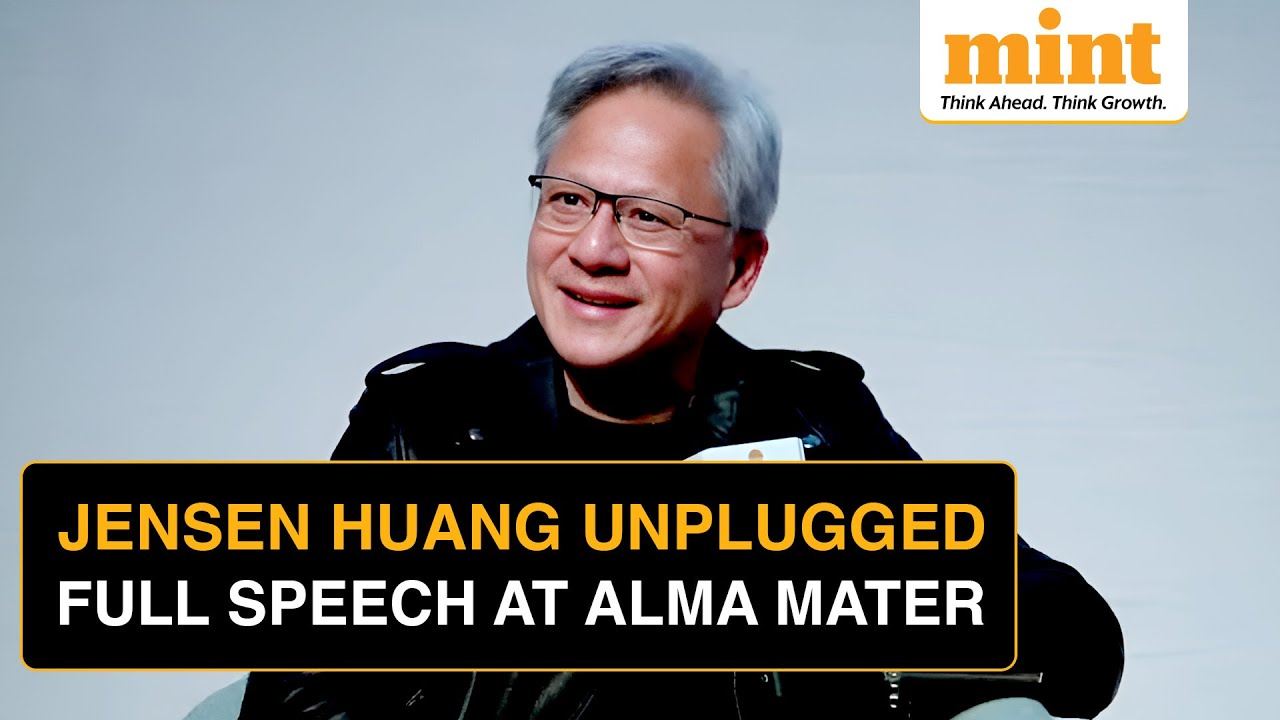
But despite all these areas of development, Huang says that we still can’t fully trust the best answers that AI can deliver. “Today, the answers that we have are the best that we can, but we need to get to a point where the answer that you get is not the best that we can provide, and somewhat you still have to decide whether is this hallucinated or not hallucinated, does this make sense, is it sensible or not sensible? We have to get to a point where the answer that you get, you largely trust.” He also added, “I think that we’re several years away from being able to do that, and in the meantime, we have to keep increasing our computation.”
Despite this, he also pointed out what Nvidia has done for AI, “What Nvidia has contributed is that we’ve taken the marginal cost of computing and reduced it by a million times.” He said, “When the cost of something reduces by a million times, your habits fundamentally change…that is the single greatest contribution Nvidia ever made, that we made it so that using a machine to learn exhaustively an enormous amount of data is something that researchers wouldn’t even think twice to do. That’s why machine learning has taken off.”
When someone pointed out that Nvidia’s AI GPUs are still expensive, Huang said that it’d be a million times more expensive if Nvidia didn’t exist. “I gave you a million times discount in the last 10 years. It’s practically free!” said Jensen.
Stay On the Cutting Edge: Get the Tom's Hardware Newsletter
Get Tom's Hardware's best news and in-depth reviews, straight to your inbox.
Jensen Huang promised his wife he’ll be CEO by 30
One other interesting tidbit that came up during the interview was when they asked Huang for advice on “when and why they should start their own thing [company].” The interviewer added, “Besides, you did promise your wife Lori that by the age of 30, you will do a company,” and Huang responded, “That was my pickup line; I didn’t mean it.”
Jensen was 17 when he met his wife, Lori, who was 19 then. He was the youngest kid in his class, and there were only three women among the students. Huang said, “You have to learn how to have a good pickup line.” So, Jensen approached her and asked, “Do you want to see my homework?” She eventually agreed since he promised that if she did homework with him every Sunday, she’d get straight As—resulting in a weekly Sunday date for Huang.
“Just to make sure that eventually she marries me, I told her that by the time I’m 30, I just wanted you [to] know I’m only 20 right now. But by the time I’m 30, I’m going to be a CEO—I have no idea what I was talking about—and then we got married.” Jensen said. “That’s all the advice I’m going to give all the entrepreneurs. That’s it.”
Jowi Morales is a tech enthusiast with years of experience working in the industry. He’s been writing with several tech publications since 2021, where he’s been interested in tech hardware and consumer electronics.
-
Math Geek jensen "still nothing really worth all the hype yet, but keep spending all that money and maybe in a few years we'll be able to do something interesting!"Reply -
hotaru251 But by the time I’m 30, I’m going to be a CEO—I have no idea what I was talking about—and then we got married.” Jensen said. “That’s all the advice I’m going to give all the entrepreneurs. That’s it.”
....so his advice to people is lie if its beneficial to you....yup sounds like a rich person's advice. -
ekio To make better AI algo quality, you need MORE gpus!!Reply
To make better music, you need more pianos too !!!
To make better buildings, you need more concrete !!!
To make better planes, you need more turbofans !!!
After the moore law, the mooron law. -
bit_user
I think it's probably not too hard to train AI to check its answers in similar ways that humans would check either an AI-generated answer or maybe that of a human we have reason to doubt. This will certainly increase the time it takes for AI to reach good answers, as it would then have to do more iteration to pass such a built-in "BS filter".Jensen said:We have to get to a point where the answer that you get, you largely trust.
I've heard that human brains have something like a "BS filter" that suppresses thoughts that don't make sense. Certain drugs inhibit this inhibitory interaction, which potentially explains why some people associate them with increasing creativity. -
hotaru251
except they can't "think" for themself if something sounds off. llm treat all data as "real" thats why "poisoning" is so bad to them as you cant untrain them like you can a human.bit_user said:I think it's probably not too hard to train AI to check its answers in similar ways that humans would check either an AI-generated answer or maybe that of a human we have reason to doubt.
it trying to check if its training is right only works if its fully human input that has been verifiably true but data learns off other databases of llm so if u fact check a lie to a lie you assume its truth. -
Gururu the ai he describes is heavily crafted by the human experience. I wonder if there are any outfits out there are approaching it the way nature would, only a billion times faster.Reply -
bit_user
This point is bogus. Furthermore, there seems to be some serious inflation of the claim, perhaps even greater than Nvidia's stock price!The article said:When someone pointed out that Nvidia’s AI GPUs are still expensive, Huang said that it’d be a million times more expensive if Nvidia didn’t exist. “I gave you a million times discount in the last 10 years. It’s practically free!”
: D
As far as I can tell, this is referring to "Huang's Law", where just over a year ago, he was claiming only 1000x speedup over 10 years.
https://www.tomshardware.com/news/nvidia-outlines-jensen-huangs-law-of-computing
The way he arrived at that figure was: 16x from better number handling, 12.5x from using reduced-precision fp and int arithmetic, 2x from exploiting sparsity, and 2.5x from process node improvements.
Most of those areas are wells you can't keep going back to. Sure, there has been further work on reducing precision and yet further improving sparsity handling, as well as weight compression and arithmetic approximations. So, I'm not saying the well of innovation has run dry. But, when you take a somewhat generic architecture and optimize it for a specific problem, a lot of the big gains are achieved early. So, I'm not expecting to see a repeat performance, here.
As for the claim of 1M, that remaining 1000x can only be referring to the scalability achieved through their HGX systems' utilization of NVLink and NVSwitch to link an entire rack's worth of GPUs into a coherent memory space. This last 1000x is perhaps the most troubling, since it only scaled somewhat linearly with cost. In other words, 1000x GPUs is going to cost you more than 1000x the price of one (assuming both are obtained through the same channel and not just comparing the retail cost of one vs. direct-sales cost of 1000). So, he provided the capability to scale, but not a real cost savings.
Briefly returning to the 1000x scaling from "Huang's Law", for a moment, a lot of these same things were being done by others. Plenty of people were looking at reducing arithmetic precision, building dedicated matrix-multiply hardware, etc. Nvidia was among the first to deploy them at scale, but it's not as if the industry wouldn't have moved at a mostly similar direction and pace, had Nvidia not been in the race.
Huang's biggest contribution was probably his aggressive push of CUDA and GPU compute and embracing the industries and applications where it was found to have the greatest potential. That's what I think gave them an early lead, down the path of AI. It's a lead they can keep, only if they're nimble, and here's where CUDA could turn out to be a liability. That's because it forces them to make their GPUs either more general than you really need for AI, or else they have to break CUDA compatibility and do a whole lot of work to adapt the existing CUDA-based software to newer architectures. This is what I think Jim Keller meant by his statement that "CUDA is a swamp, not a moat". It's now become an impediment to NVidia further optimizing its architectures in ways similar to its biggest AI competitors (who, other than AMD and Intel, generally seem to have embraced data-flow architectures over classical GPUs). -
bit_user
Why can't it check a claim the same way you would? Why can't it do some internet searches and look at sources to confirm?hotaru251 said:it trying to check if its training is right only works if its fully human input that has been verifiably true