New AI accelerator slots into an M.2 SSD port — MemryX launches $149 MX3 AI Accelerator Module capable of 24 TOPS
The module is easy to deploy into any system with a PCIe Gen 3 M.2 slot
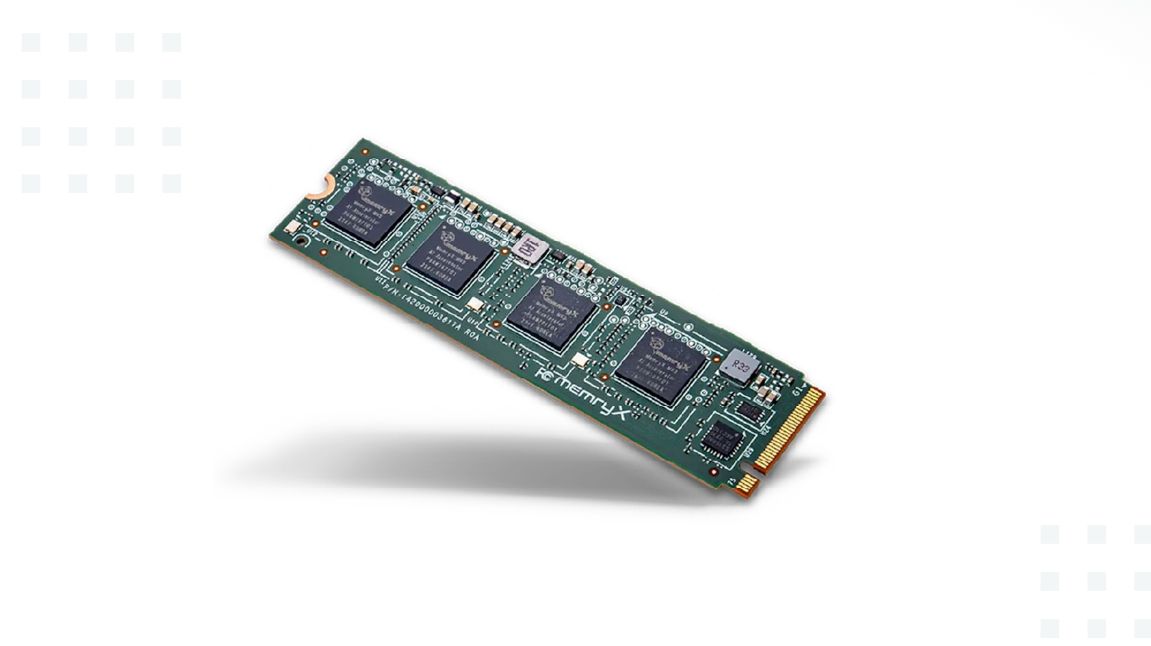
MemryX, a technology start-up originating from the University of Michigan, has launched a $149 M.2 module [PDF] designed to provide efficient AI processing capabilities for compact computing systems. The module targets edge computing applications, where power efficiency and compact design are critical.
Packing four MemryX MX3 AI accelerator chips onto a standard M.2 2280 form factor, enables the module to be easily integrated into systems equipped with a PCIe Gen 3 M.2 slot. Each MX3 chip is said to deliver 6 TOPS (Tera Operations Per Second), offering a total of up to 24 TOPS of compute power while consuming just 6 to 8 watts of power. It also supports a range of data formats, including 4-bit, 8-bit, 16-bit weights, and BFloat16. Notably, the module operates without active cooling, relying on an included passive heatsink to manage thermal performance.
Phoronix has tested the MemryX MX3 M.2 module, evaluating its performance and usability as an AI accelerator. Installed in a system with an M.2 PCIe Gen 3 slot running Ubuntu 24.04 LTS, the module proved straightforward to integrate thanks to MemryX's open-source drivers and developer tools. The module's 24 TOPS performance from its four MemryX MX3 chips is said to be sufficient for various inference workloads, particularly those optimized for 8-bit weights.
The review also highlighted the module's strong software compatibility, supporting frameworks like TensorFlow and ONNX, and its efficiency in running small to medium-sized AI models. Each MX3 chip supports up to 10.5 million 8-bit parameters, with the module's four chips combined handling a total of up to 42 million parameters. This limitation arises from the absence of onboard DRAM. MemryX plans to address that with a new PCIe card in 2025 that will feature more MX3 AI chips onboard.
The MX3 M.2 module is available for $149 USD, making it an affordable option for developers and organizations seeking to add AI processing capabilities to edge devices. MemryX has also announced that the module will be showcased at the upcoming Consumer Electronics Show (CES) 2025 in Las Vegas where it plans to demonstrate the MX3's performance across various real-world applications, further highlighting its versatility.
Stay On the Cutting Edge: Get the Tom's Hardware Newsletter
Get Tom's Hardware's best news and in-depth reviews, straight to your inbox.
Kunal Khullar is a contributing writer at Tom’s Hardware. He is a long time technology journalist and reviewer specializing in PC components and peripherals, and welcomes any and every question around building a PC.
-
bit_user This thing is virtually useless, for most users. It won't run LLMs or Stable Diffusion. And no, adding two of them won't turn your machine into a "CoPilot+" PC.Reply
The main problem it faces is the lack of DRAM and connectivity is too poor for it to effectively fall back on host memory as a substitute. Even with PCIe 5.0, I think that would be an inadequate solution. -
scottscholzpdx
25 TOPS is enough for text-response AI, even with slower memory.bit_user said:This thing is virtually useless, for most users. It won't run LLMs or Stable Diffusion. And no, adding two of them won't turn your machine into a "CoPilot+" PC.
The main problem it faces is the lack of DRAM and connectivity is too poor for it to effectively fall back on host memory as a substitute. Even with PCIe 5.0, I think that would be an inadequate solution.
Sounds like someone has a horse in the race. -
bit_user
What good is 25 TOPS when you're limited to streaming in weights at a mere 4 GB/s (best case)? That PCIe 3.0 x4 interface would likely be such a bottleneck that you might as well just inference on your CPU, if your model doesn't fit in the on-chip memory.scottscholzpdx said:25 TOPS is enough for text-response AI, even with slower memory.
Furthermore, their datasheet doesn't even say it's capable of weight-streaming from host memory! It just says it supports models with 40M 8-bit parameters (or 80M 4-bit), period.
Most pointedly, they themselves don't even list LLMs as among its possible applications. The only demo I've seen is object detection/classification.
I point out lots of weaknesses in products written about on this site. That doesn't mean I have a stake in competing products.scottscholzpdx said:Sounds like someone has a horse in the race.
I found the article alarming, because the author seems to be essentially regurgitating a press release and utterly failed to point out critical weaknesses and limitations in the product. It's so bad that I found myself questioning whether the author even understands what they're writing about or how an end user might even use it. The reason for my comment is simply to warn others about these oversights.
Are you really so confident it can be used for LLM inferencing that people should just go ahead and buy it? Did you even look at the datasheet of the product? If not, you might want to do that, before replying.
https://memryx.com/wp-content/uploads/2024/11/MX3_M.2-Edge-AI-Module-Product-Brief.pdf -
kuramakitsune
Microsoft itself says that 40TOPS is what it considers to be the minimum for AI loadsAdmin said:The MemryX M.2 module delivers efficient AI acceleration for edge computing with 24 TOPS of performance, low power consumption, and seamless Linux integration
MemryX launches $149 MX3 M.2 AI Accelerator Module capable of 24 TOPS compute power : Read more -
purposelycryptic
Maybe for your purposes, but for anyone currently using a Google Coral TPU, which, given how long every version of it was consistently sold out for, is quite a few, this would present a fairly significant boost.bit_user said:This thing is virtually useless, for most users. It won't run LLMs or Stable Diffusion. And no, adding two of them won't turn your machine into a "CoPilot+" PC.
The main problem it faces is the lack of DRAM and connectivity is too poor for it to effectively fall back on host memory as a substitute. Even with PCIe 5.0, I think that would be an inadequate solution.
I personally use a dual Coral TPU card in my home automation server to handle real-time security camera footage processing, since I the idea of sending it to some company's cloud is all kinds of disturbing to me, and it does a great job with facial/license plate recognition, etc.
Point is, there are all kinds of uses for this kind of thing other than just running local LLMs, and it has more than enough processing power for many people's purposes. -
bit_user
A lot of cameras now have that capability builtin, even cheap ones. A friend mentioned that he recently bought some $40 cameras and he thought their object detection & classification capability is pretty good.purposelycryptic said:I personally use a dual Coral TPU card in my home automation server to handle real-time security camera footage processing, since I the idea of sending it to some company's cloud is all kinds of disturbing to me, and it does a great job with facial/license plate recognition, etc.
Speaking of price, the dual-processor Coral board is only $40, while this board is $149. They say there's a version with only two chips, but I don't see it for sale. This offers 6 TOPS per chip, while Coral offers only 4. This seems to have 10 MB of weights storage, while Coral has 8 MB. So, it's better than Coral, but also nearly twice as expensive per chip. However, Coral launched 5 years ago, so I'd say the MX3 isn't nearly where it should be, which is about 10x as good as the Coral and costing roughly the same.
I think there's also a possibility the MX3 won't support some layer in the model you might want to use, so that would be another thing to check. Google is very clear about which layer types Coral supports, and you can use it via TensorFlow Lite.
I would say not "all kinds", due to the severe model size limitation. For larger models, Coral can stream in weights, whereas nothing in the MX3 literature indicates it can do the same.purposelycryptic said:Point is, there are all kinds of uses for this kind of thing other than just running local LLMs, and it has more than enough processing power for many people's purposes.