Research shows more than 80% of AI projects fail, wasting billions of dollars in capital and resources: Report
The AI industry is fraught with failure.
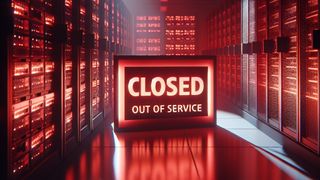
AI is currently one of the hottest topics for those looking to invest in “the next big thing”. But according to research by the RAND Corporation, over 80% of these AI projects will fail — which is twice the failure rate for non-AI technology-related startups. The global policy think tank talked to 65 data scientists and engineers who have been working in the artificial intelligence sector over the past years, and they’ve determined several causes that lead to this massive failure rate.
According to the research, the biggest reason for the failure of AI projects is the misalignment of goals between key stakeholders. The leadership often has a view of what AI can and should achieve that is not grounded in reality; instead, it’s driven by humanity’s pre-conceived notion of what AI is, most often fuelled by Hollywood. This lack of understanding between business leaders and the people on the ground means that projects often do not have the resources and time needed to accomplish their goals.
However, the engineers working on the sharp end of AI aren’t blameless, too. The interviews revealed that data scientists sometimes get distracted by the latest developments in AI and implement them in their projects without looking at the value that it will deliver. This “shiny object syndrome” means that the scientists and engineers want to use these new technologies just because it’s the latest development. While it’s important to stay up to date on AI, teams should also consider whether that new tech would actually solve the problems they face in their research, or if it would only make it more complex and convoluted than it already is.
There are also several other reasons noted in the research, including the lack of properly prepared data sets, inadequate infrastructure, and the incompatibility of AI to the problem at hand. It also noted that these problems aren’t limited to the private sector: even the academia have issues with AI projects, where many focus simply on publishing AI research instead of looking at real-world applications for their output.
This research is proof behind the many consolidations and failures we see in the AI industry. In fact, Baidu CEO Robin Li Yanhong said China has too many large language models and that they’re wasting a significant amount of resources because these often have few, if not zero, practical real-world applications. We can also see this with the number of generative AI patents that China has filed in the past decade, outpacing the U.S. 6-to-1. But despite that, only one Chinese organization, the Chinese Academy of Sciences, made the top 20 entities that received the greatest number of citations between 2010 and 2023.
The rush to get ahead in the AI race is making many companies act a little rash in building their AI projects. While they (and their investors) are the only ones who bear the risk of any failed project, it would still be wise for them to look carefully at the failure of other AI projects and the reasons behind it. After all, if AI projects fail to deliver their promises over a long period, then the entire industry could fall and burst like a trillion-dollar bubble.
Stay On the Cutting Edge: Get the Tom's Hardware Newsletter
Get Tom's Hardware's best news and in-depth reviews, straight to your inbox.
Jowi Morales is a tech enthusiast with years of experience working in the industry. He’s been writing with several tech publications since 2021, where he’s been interested in tech hardware and consumer electronics.
-
chaz_music AI and neural nets have been around for DECADES. When I was in grad school, we had all sorts of names for what we call AI: neural networks, fuzzy logic, adaptive control, adaptive observer, etc. These systems have been used for things like trajectory guiding and automobile cruise control for many years. The only thing new now is that we have the ability to connect many more nodes than before, and it has been made available to the public to play with making pictures and term papers. And available to people to do great harm.Reply
If AI was a sure thing, big tech like IBM and Microsoft would have developed it many many years ago. The sure thing is the end application such as medical devices, drugs, and other critical applications. Another is crunching large data sets to get a significant finding, like finding an asteroid that is going to strike the earth in a few solar cycles. But these take people who are close to the end application to utilize AI properly and make the esoteric models for the AI to learn correctly. So teach it "Here are the rules, now go find me a dangerous asteroid using the telescope data."
All it takes is for someone to give a new name for something old and it turns into a fad, draining tons of resources from the venture capital pool that would have otherwise been supportive for other great start up tech. Example: Digital Twin. This is simply making a viewable physical model attached to an already existing mathematical model = "simulation" in the VR world. We have been able to create viewable models in the mechanical CAD world for at least 20 years or even more. But Digital Twin marketing is allowing companies to make it seem as though we have "new" technology and raking in tons of cash. Makes me think of the pet rock fad. -
Gururu It'd be nice if this "waste" was trickling down to the middle and lower classes and not making a few instant millionaires.Reply -
vanadiel007 You mean talking to ChatGTP is not making money? Who would have thought.Reply
No worries, Nvidia et all has you covered with tons of new GPU solutions arriving at a regular interval.
They are often sold out, so you better place your millions dollar order right now to avoid not getting those fancy GPU's. -
kanewolf According to the National Restaurant Association, 80% of restaurants fail within 5 years. The U.S. Bureau of Labor Statistics (BLS), says that approximately 20% of new businesses fail during the first two years of being open, 45% during the first five years An 80% failure rate is not surprising.Reply -
Giroro Nobody has the slightest idea how to use their shiny new billion dollar super computer to make enough money to pay for itself.Reply
That's eventually going to a a big problem for everybody. If you think AI is annoying now, just wait until some of these companies start desperately trying to squeeze you for cash, to try and pay off their investors.
It probably will even be a big problem for Nvidia, unless they're always being paid up-front, in cash. -
shtldr
You could have YOLO'd your life savings on Nvidia stock call options, like some d3g3n3r4t3s on r/wallstreetbets, and become a millionaire, too. Or did you expect people to become rich while taking zero risk and just standing by?Gururu said:It'd be nice if this "waste" was trickling down to the middle and lower classes and not making a few instant millionaires.
There are going to be many AI investors (including the biggest corporations), who will lose a lot of money in the end (FoMO investing into AI without a proper use case). Would you like these losses to trickle down to you, too? :D
Every bubble is a zero sum game. If you don't play, you don't win (or lose). -
edzieba The "80%2 figure is not from some deep analysis, the source they cite is just some op-ed piece. And with general failure of startups being between 75% and 90% depending on who you ask (and what industry, and what country, etc), that figure would not be out of the ordinary even if accurate.Reply
There is a little more to a Digital Twin.... but only a very little. A proper Digital Twin is a simulation running in parallel with the actual hardware (an assembly line, a building, etc) with the outputs of that simulation compared to sensor measurements of the actual environment to flag up "hey, something is not happening like its supposed to". Basically picking out the error term of a closed-loop feedback system but taking the system-of-systems approach rather than only applying it to subsystems.chaz_music said:Example: Digital Twin. This is simply making a viewable physical model attached to an already existing mathematical model = "simulation" in the VR world. We have been able to create viewable models in the mechanical CAD world for at least 20 years or even more. But Digital Twin marketing is allowing companies to make it seem as though we have "new" technology and raking in tons of cash. Makes me think of the pet rock fad.
But yes, 99% of 'digital twins' sold to businesses are just "here's a copy of the CAD for your building plans, go update it to the as-build state and then keep updating it" that is never updated and is left in a drawer somewhere never to be looked at again, because the buyer has no clue what a Digital Twin actually is or why they want one other than buzzword-chasing, and there are plenty of unscrupulous 'consultants' happy to sell a company their own CAD files back to them at a tasty markup.
When everyone figures out that Large Language Models don't have any actual utility to anyone and a bunch of accelerators are offloaded at bargain basement prices on ebay, the real winners will be those who have been training specific-task models for decades (stellar object classifiers, crop status monitors, etc) who now have cheaper iron that run faster. -
Amdlova The drug dealer don't use drugs.Reply
Nvidia is top seller of dreams.
Crazy mining, Crazy AI... just Tons of money... some day We will grab this enterprise hardware cheap on ebay.
Can it run crysis. -
kealii123 Good LLMs like GPT4 is definitely disrupting the software development industry. I use copilot every day, and despite the fact that its a crappy MS product (I often just screenshot paste directly into GPT4 instead) I'm probably twice as fast. Better products like Cursor can allow an 8 year old child to program faster than a CS college freshman on their own:Reply
1825581674870055189View: https://x.com/rickyrobinett/status/1825581674870055189?t=pevnj7taM3r8oabugoq4hA&s=19
Iterative, self-reinforcing AI coding projects like Devin show that a good LLM can be as productive as a junior engineer if allowed. If you take Llama3 or the latest Claude and train it on your repos every night, then allow it to edit code & iterate on the outcome, I bet you could get mid level, SE2 performance out of it with less than the annual salary worth of hardware.
Once the cost of writing software collapses, anything software-dependent (everythnig) will also shrink significantly in price and innovation will grow dramatically.